Simple & Pretty – Product design & strategy for startups
Managing a beta program for a pool safety product powered by AI
Beta testing is a great way to conduct user research and gather feedback on your product before launch. However collecting, analysing and actioning the data can be very time consuming and resource intensive without a clear purpose.
This is how I did it as a UX research team of one.





While working as lead UX researcher for Deep Innovations, the company rolled out a beta program for PoolScout, its flagship product, a new pool safety system that uses advanced AI to identify and distinguish toddlers from adults and pets, in and around the pool, to provide timely alerts. The system includes an outdoor IP camera, an alarm unit and a cross-platform mobile app.
Two were the main goals set for the beta:
1.To gather real-life, video analytics data for the AI team to improve the algo performance before market release
2.To evaluate PoolScout with pool owners and unveil any critical issues needed to be fixed (with the hardware, the technology or the app) to increase market fit opportunities
For months before kickstarting the beta program, the product team worked towards finding the right balance between sharing PoolScout early enough with testers to gather priceless feedback, while ensuring the system was functional and stable enough not to frustrate them. This was particularly needed due to the nature of PoolScout, being a safety product powered by AI. Launching an immature product without warning would be dangerous and result on lack of trust in the "intelligence" of the Algo and the accuracy of its classifier.
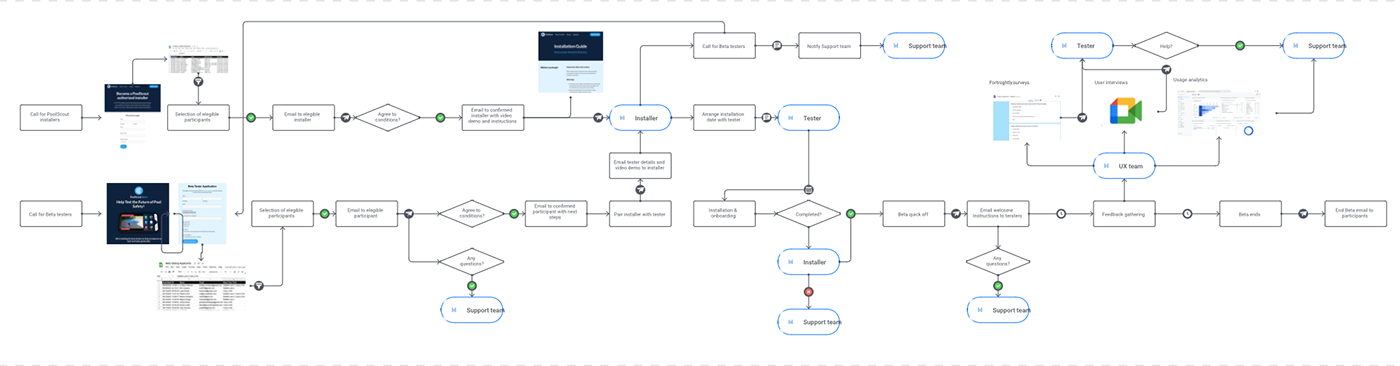
Beta program blueprint
Setting realistic expectations and valuing testers' participation
To establish trust and positive rapport with the beta participants, we openly shared with them purpose, key objectives of the program and where the product was in terms of functionality and usability. Communicating clearly the context and limitations of PoolScout as a product in beta phase, helped setting expectations with participants so they could provide feedback that was relevant and useful.





We highlighted the value of their contribution and thanked them in advanced. Testers were incentivised upfront with the hardware kit with professional installation, and once the product launched, 12 months of subscription.
Acknowledging the importance of testers' role and rewarding them for it lead to a wider sample of engaged and motivated participants. Knowing that their participation could help to shape the future of pool safety was also a key motivator to take part in the research, leading to a larger and more representative sample size.
Providing multiple ways to share feedback
Over 200 pool owners across the USA participated, during 12 months. They shared regular feedback on PoolScout via interviews, surveys, in-app feedback forms, report 'False Alert' in-app functionality, and emails and comms with Research, Support, Marketing and Sales teams. Participants regularly shared videos and app screenshots for the product teams to analyse and action.
In addition, we regularly monitored Google and Apple analytics to track app usage and performance, and to identify any significant trends that we could explore deeper with qualitative research and further testing.
As a result, sharing their feedback was accessible to testers with different preferences, needs and abilities, making it easy and convenient to participate in the research, and leading to more inclusive and diverse feedback.




A dedicated space to collect, organise, analyse, share and report
Within Jira, the project management software used in the company, we created a 'Beta feedback' project for gathering and organising the data. This enabled us to identify, prioritise and action issues efficiently within the same tool. Working closely with business stakeholders and the product team allowed us to determine how to best incorporate into the product development before launch.
When possible, we used automations to speed up the process of feedback collection. We used Jira collectors in the app's forms, web hooks to receive the data from the feedback surveys submitted in our website and calendar integrations to arrange interviews with beta participants.
As a minimum, each Jira issue included a summary, the feedback type (observation, confusion, positive/negative feedback, feature request, bug), the feedback source (interview, email, in app form, survey...) and the related epic. Epics where used to associate issues to the key objectives of the beta: Improving Algo detections, safety alerts, livestream performance, app usability, the system's hardware (camera & siren) and the end-to-end customer journey.


Tagging and grouping feedback to analyse and report findings
Some Jira tickets on their own unveiled major issues, but more commonly, were when grouped into trends and patterns, that these single pieces of feedback reveal stories (insights) where friction was occurring for the many, and where opportunities for improvement really were.
To further analyse the feedback, we set up customised fields, components and labels to tag different pieces of content. we used issue status to help categorise and organise the data, making it easier to review and synthesise. Seeing at the top level the statuses "Feature request", "Positive feedback", "To analyse", "Urgent to action" "In progress" and "Done", allowed the product team to easily find and access relevant information and take action when needed.
This increased collaboration and sharing enabled stakeholders such as the CEO, CTO, head of design, software lead, Support, Marketing and Sales teams to share and collaborate on research findings, promoting cross-functional collaboration and more informed decision-making. It also enabled the product team to track the research findings over time, allowing for more accurate and transparent reporting on progress and results, ensuring that research was always relevant and actionable.



The ROI of user research
As a result of the feedback collected, analysed and shared during the beta, many improvements and important fixes were done to PoolScout before launch.
The product team quickly tackled pool setup and algo misclassification issues by including 'Activity Zones' and 'Installer mode" in v1, two features that were planned for later in the roadmap. The software team significantly improved the video player's latency and connectivity fixes to the livestream. The hardware team released several camera and siren firmware upgrades to fix false positives generated by objects and weather conditions. Design, customer success and sales teams iterated, filled the gaps in the process and flows, and improved customer onboarding, setup, installation and support.
Conducting the beta program with real pool owners before launch, enabled the PoolScout team to identify and fix many issues before market release. This saved the business unknown extra time and money on post-launch fixes and customer support. It also dramatically improved the experience for all users and helped the team to identify new opportunities for product development, which will lead to more savings and new revenue streams.
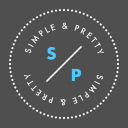